Intelligent automation is the foundation for building up your digital transformation strategy. It’s more than just going paperless or eliminating slack in a process — it’s how organizations can deliver great service, both within their own teams and for their customers. Intelligent automation is the key to connecting today’s workforce to the future of work, and if you don’t have an intelligent automation footing and roadmap for growth, you’re already falling behind.
What is intelligent automation?
Intelligent automation (IA) describes a large category of technology solutions that automate processes that were once manual, so businesses can anticipate the needs of users and customers. It helps organizations simplify or eliminate tedious tasks through technology that learns as it operates, meaning the product will work better six months after implementation than it did after the first week, and will surpass the efficiency of the six-month mark 12 months after implementation.
What does it provide?
Intelligent automation provides a reduction in human-first touchpoints, a more digitized work environment, enhanced accuracy, a more empowered workforce and organizational resiliency. It allows your organization to process its data faster and more accurately than with a human-only workforce. According to a 2021 Forrester report, Intelligent Automation’s Value Spreads Beyond Cost Savings, “intelligent automation can free up $132 billion in economic value,” in the U.S. alone, including:
- $68 billion among cubicle workers
- $44 billion among coordinators
- $18 billion in function-specific knowledge workers
- $23 billion single-domain knowledge workers
What provides intelligent automation? Many organizations capitalize on their intelligence strategy by leveraging the embedded intelligence within their foundational technology platforms. For example, leading content services platforms (CSPs), increasingly provide intelligent content services, which means IA tools like artificial intelligence (AI), machine learning and advanced analytics are native capabilities to the CSP. While still a burgeoning trend, the use cases for embedded intelligence within content services is seen as a transformational opportunity in the industry.
Why is it important to intelligently automate?
Data is being created in our world at staggering rates: “On average, information volume coming into organizations is expected to grow by 4.5X over the next two years, with nearly 60% of that information to be unstructured (like a contract or a conversation) or semi-structured (like an invoice or a form),” according to AIIM. How — or whether — we harness and use that data is the question of the moment. The same AIIM report discovered a cumulative D+ grade for organizations’ ability to “extract intelligence from information.” A tsunami of data coming into your organization without the ability to quickly and accurately recognize it, extract it and organize it puts your team at a loss, with impacts such as:
- Inability to see the whole/entirety of your data, diminishing business intelligence
- Competitive disadvantage when compared to savvier users of intelligent automation
- Underwhelming business performance
- Unsatisfied customers
- Overworked, undervalued, disengaged and frustrated employees
How does intelligent automation work?
Intelligent automation success, no matter which tool is being considered or deployed, is predicated on the prework that went into designing the solution. In previous generations of technology, deployments may have begun with an identified technology, but in today’s fast and fluid business structure, one size doesn’t fit all. The first step in pursuing an intelligent automation project is a comprehensive data-gathering mission centered around the business challenges you want to solve. Ironically, your success with intelligent automation begins with people. Once the preliminary implications and desired outcomes are identified, you need to choose a partner to help guide you to the best-fit solution. Any intelligent automation solution, regardless of which tool is chosen, will need to:
- Integrate with your other line-of-business systems
- Predetermine criteria, tasks, relationships and related actions, and encode them into machines
- Execute pre-built rules, such as “if-then” scenarios and confidence-level programming
- Continuously improve its functionality through what it’s learned during operation
Intelligent automation vs. RPA
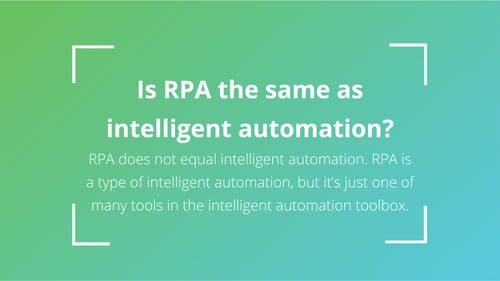
Intelligent automation tools
Intelligent automation tools deploy a range of strategies to reduce the amount of human touch required in business processes. With infinite types of job tasks across industries and in various departments, there are many tools that have been designed to automate those processes effectively. The tools in the intelligent automation toolbox are dynamic and diverse, ranging from autonomous (no human interaction required) to semi-autonomous and even human-led, such as a workflow tool that is executed by the user.
Machine learning
Machine learning (ML) is a subcategory of AI where a computer uses algorithms and statistical models to learn how to perform specific tasks without the need for instructions from a human-user. ML uses patterns and inference to complete tasks. Each time an ML process runs, the system can use the results to measure the algorithms’ accuracy and make improvements automatically. Read more about embedded ML. Deep learning Deep learning is a subset of machine learning, and it’s the behind-the-scenes training that teaches computers to learn like a human brain. This is how computers learn to recognize patterns and identify unstructured data, through continued exposure to different content and deep learning feedback.
Intelligent capture and extraction
When data arrives to your organization, intelligent capture and extraction tools automatically ingest that data using pattern recognition (not templates) to recognize and interpret the information on a document. It begins its automation journey by learning what kind of data it should expect by processing just a few sample pages of the forms you’re using and begins to understand the different document types and data locations on each.
Optical character recognition - Optical character recognition (OCR) is an intelligent capture tool that extracts data from a scanned document or image file and converts the text into a machine-readable format for use in data processing. Organizations that employ OCR to convert images and PDFs (typically originating as scanned paper documents) free up human resources and save time that would otherwise be spent managing unsearchable data.
Optical character recognition - Optical character recognition (OCR) is an intelligent capture tool that extracts data from a scanned document or image file and converts the text into a machine-readable format for use in data processing. Organisations that employ OCR to convert images and PDFs (typically originating as scanned paper documents) free up human resources and save time that would otherwise be spent managing unsearchable data.
Optical mark recognition - Optimal mark recognition (OMR) determines a selection from a list of choices, such as check boxes and filled circles.
Bar code recognition - Bar code recognition (BCR) extracts data from bar codes on the document.
Process automation
According to Deep Analysis, business process automation orchestrates multiple complex and parallel activities and is often used as the cornerstone to integrate multiple data stores, applications and people. Implementing a content and process automation use case, like claims processing or new account onboarding, requires strong workflow and content intelligence capabilities that integrate with core line of business applications.
Low-code development tools
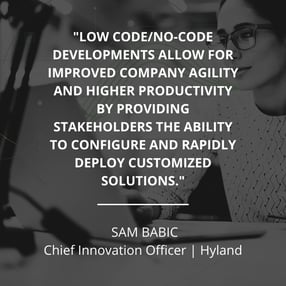
Natural language processing
Natural language processing (NLP) is the technology within machines that understands the way we communicate. It accumulates data points and feedback as it is used, puts context around the way we express ourselves and leverages implied knowledge to produce high-quality results. This use of implied knowledge creates the most likely and natural results based on what the machine knows about our needs. For example, when a document with unstructured data comes into your system, NLP identifies the content for contextually relevant terms, transforming the data from unstructured to structured.Intelligent automation trends
The technology industry is fast-moving with digital transformation trends coming and going, and new solutions entering the market all the time. Sometimes the newest concept takes off, finding its way into organizations quickly and to great success. Other times, exciting new concepts see slow but steady adoption, eventually finding their place into the everyday. And for some concepts, early promise leads nowhere. Here are the intelligent automation trends to keep on your radar.
Embedded intelligence
Embedded intelligence describes components of AI that are native to a platform. For example, a content services platform with embedded intelligence can predict, classify and enrich content based on business-specific needs using its foundational capabilities. Although AI has long been table stakes for CSPs, the market is evolving to demand that intelligent features be embedded in solutions. Market trend: In the 2021 Gartner® Magic Quadrant™ for Content Services Platforms, Gartner noted embedded intelligence as one of four key trends impacting content services, and said: “AI is critical to content services. In the past, it has been an interesting feature looking for a use case. However, it is becoming increasingly embedded with real business solutions from correspondence management to case management.”
Deployment in the cloud
Deploying your IA solutions in the cloud still qualifies as a trend — but just barely. The tactic is quickly becoming mainstream for businesses, with 13% of organizations in a Deloitte survey already running their automation solution solely in the cloud, and nearly half already using it for at least some automation. Cloud deployment helps organizations scale faster and better accommodate growth and changing business conditions. It also:- Streamlines the management of your solutions
- Allows experts to manage and optimize your platform’s IA tools for increased project success
- Frees up IT resources so they can focus on achieving goals instead of managing infrastructure like patches, security configurations and upgrades
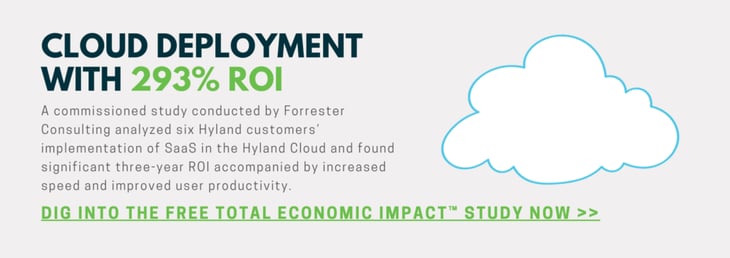
Hyperautomation
Based on the need for a more coordinated, wider-reaching automation approach, many organizations are adopting the idea of hyperautomation. “Hyperautomation is a business-driven, disciplined approach that organizations use to rapidly identify, vet and automate as many business IT processes as possible,” according to Gartner. This push typically deploys a variety of intelligent automation tools, especially AI, ML and RPA to get as many processes as possible running without human intervention. Market trend: Gartner named hyperautomation a tool for sculpting change in its Top Strategic Technology Trends for 2022 and noted: “By 2024, diffuse hyperautomation spending will drive up the total cost of ownership 40-fold, making adaptive governance a differentiating factor in corporate performance.”Intelligent automation examples
The impact scenarios for intelligent automation are endless, but we know real-world applications go a long way in truly seeing how a solution can improve the reality for your people and processes. Here are three intelligent automations examples and how the technology can impact an organization. Looking for more examples? Read 5 examples of what intelligent automation could look like at your organization.
A clothing manufacturer deploys AI and machine learning
The problem: A company that manages several clothing brands realized the same white T-shirt was being photographed by multiple groups within the company, multiple times a year, without anyone realizing it. The images didn’t have metadata, and the redundancy was wasting time and resources. The solution: Implementing AI and ML tools to an existing digital asset management (DAM) system launches a process that extracts information from images and applies the metadata in a consistent way, such as “T-shirt,” brand label, SKU, the seasonal campaign, the model wearing it and more. Read the full case study, 32 T-shirts: The importance of image metadata .Financial Services Firm uses RPA to eliminate hours of manual labor a day
The problem: Firms in the financial service sector see massive amounts of sensitive data entering their post room and mail inbox every day, in many different formats. Manually sorting these large volumes of incoming mail and routing it to the correct departments often puts pressure on the operational efficiency of organisations. The solution: The RPA solution integrated with Optical Character Recognition and a document management software to automatically trigger workflows that will route inbound mail to the correct employee. Instead of devoting valuable worker time to reviewing incoming forms, invoices and other documents to route them to the appropriate destination, this document recognition and routing technology reduced labour costs and delivers accurate results, faster. Read the full case study, Leading Financial Service Firm Digitize Their Mailroom Using Workstream RPA
Montgomery Transport Group reduces processing time by 50% with AP automation
The problem: The company’s accounts payable processes were slow and resource heavy processing up-to 200,000 invoice pages each year and resulted in slow and labor-intensive processes. That kind of lag is bad for the customer experience and bad for team morale. The solution: An AP solution leveraged intelligent capture, extraction intelligence and workflows; all invoices, no matter where they originate in the group, or what format they take, are captured by this system. These captured savings resulted in a 50% reduction in invoice processing time and the freeing up of 2.5 Full Time Employees who are free to focus on higher value activities. Read the AP automation success story.
Identifying an automation opportunity
It’s important to determine where intelligent automation solutions are best suited for your organization. Most organizations start with process mining, an exercise that uncovers the reality of existing processes and not just what processes should be or are assumed to be. This in-depth review provides a holistic understanding of your processes and identifies opportunities for automation optimizations, via technology and re-engineering. ML and AI, for example, are powerful and complex tools, but for the majority of intelligent automation projects, they bring more intelligence than needed. This can negatively impact implementation, costs and adoption. For the many automation projects that have fixed parameters, only to be changed when you decide to do so, a simpler form of intelligence is a better fit. Not every automation project requires the pinnacle of AI. Here are simple parameters: Minimally complex: Scanning, data entry, retrieving and routing- Intelligent capture
- RPA
- Natural language processing
- Federated search
- Workflow capabilities
- ML
- AI
- Skilled workers with specialized expertise
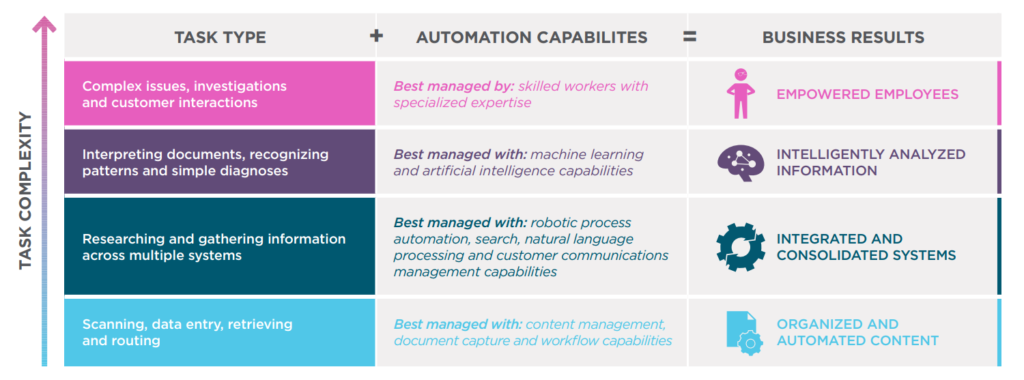
Roadmap for getting started
At the onset of your intelligent automation journey, it may seem the first step should focus on an existing technology audit. That, however, would be a misstep. Alan Pelz-Sharpe, founder of the analyst firm Deep Analysis and host of the webinar, Thinking intelligently about automation, says it’s critical that you begin with people. “Too many projects start by keeping automation projects under wraps because they’re worried people aren’t going to like it,” Pelz-Sharpe said in the webinar. “Leaders tell the managers, they talk to the supervisors, but they don’t talk to the people who actually do the job. You need to value human intelligence. Your intelligent automation project will be fed by the insights of the people in your organization.” Because your project scope — and its success — is dependent on the accuracy and fullness of the information you feed it, you need to collect data from all areas and all angles. Your automation project does not begin with buying a solution, but rather with identifying what you want to do and how you can best achieve it with the array of intelligent automation tools available. Here is a brief look at the seven steps to making automation decisions, as noted by Pelz-Sharpe in more detail in his webinar:- Analyze your organization’s existing business
- Use process mining tools, when available, to get the full picture
- Map the process journey from both the human-involved side and system-centered side
- Design your to-be state, and don’t limit your big ideas
- Recognize the full picture of people, processes and technology that will be involved
- Get advice from a community that looks like you
- Focus on value for the long term